Have you ever had a situation where you have 2 headlines and you don’t know which one to choose? Or, how about you have 2 pricing but don’t know which one people would want?
This calls for A/B testing and it does just that! It helps you test out your different ideas and see which performs the best!
Here are the key topics we’ll talk about:
- What is A/B Testing?
- Why do we do A/B Testing?
- How do you perform an A/B test?
- The Concept of A/B Testing
- A/B Testing Examples
- A/B Testing Tools
- A/B Testing, Multivariate testing, split testing
- When not to do A/B Testing
- A/B Testing Statistics & Data Science
What is A/B Testing?
A/B testing is a marketing experiment to compare and test several variations, e.g. a headline, visual, copywriting, to determine which performs better.
It is done by splitting the audience equally to reduce bias and success is measured by the number of interactions and conversions.
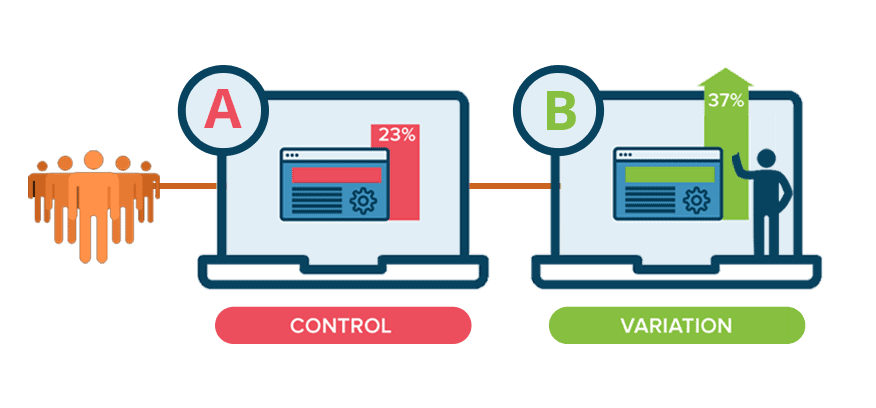
Why do we do A/B Testing?
We often have many ideas, but we are not sure what our audiences truly resonate with; which is why, we use A/B testing as a marketing experiment to determine which idea or variation works best.
A/B testing allows you to experiment on copy, video, visuals, testimonials, headlines, layouts, user experience (UX) and other elements. Sometimes a simple change can create a huge impact on conversion rates which would ultimately affect the bottomline.
Here is what a friend from AirAsia said (AirAsia is Asia’s biggest budget airlines):
We A/B testing a lot as even small variation could impact our bottom line (profit) upwards in the scale of millions.
It could be as simple as changing the colour of the button or the text on Call-To-Action. To remove the guess works out of which color, text or visual performs better; A/B testing is used for this purpose.
How do you perform an A/B test?
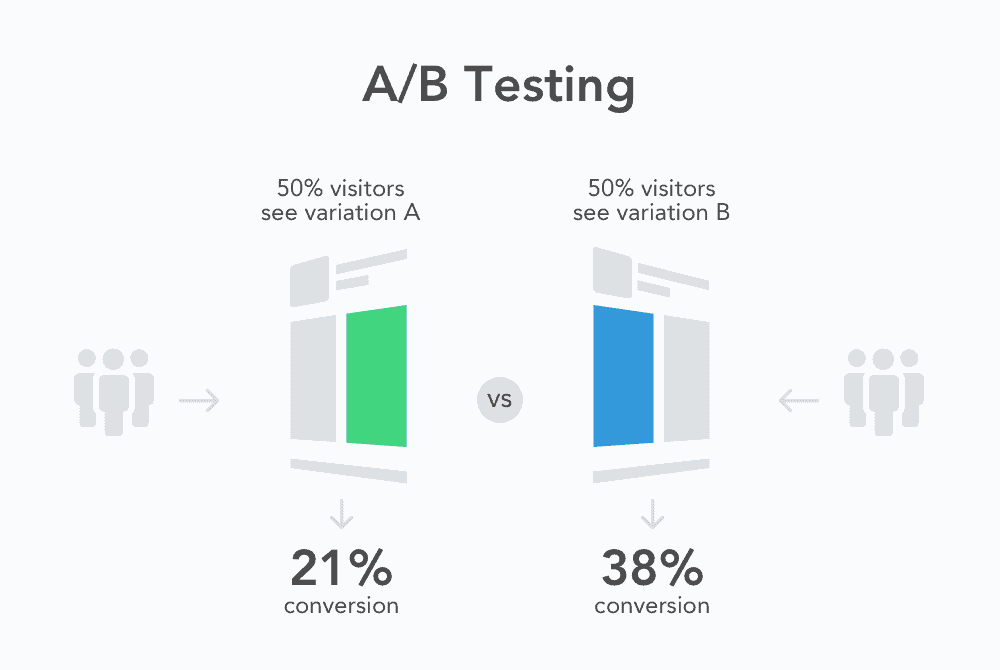
The Concept of A/B Testing
- You have 2 variations of an element which you want to test while everything else remains the same
- You split the experiment audience into 2 groups where 50% of them will see variation A, another 50% will see variation B
- Understand what is your conversion metric: is it open rate? Click through rate? Sign up rate? Purchase rate? etc.
- To measure success, you want to achieve results that are not just indicative BUT conclusive. You will need to achieve a minimum number of conversions.
Basically, you cannot run an experiment of 20 people and assume that the results will be conclusive for 1000 people.
With Facebook advertising, they suggest 50 conversions over a period of 7-days for them to move past the learning phase and choose the winning ad to focus on.
A/B Testing Examples
Here are some scenarios/ examples on how A/B Testing can be conducted.
Email Open Rate
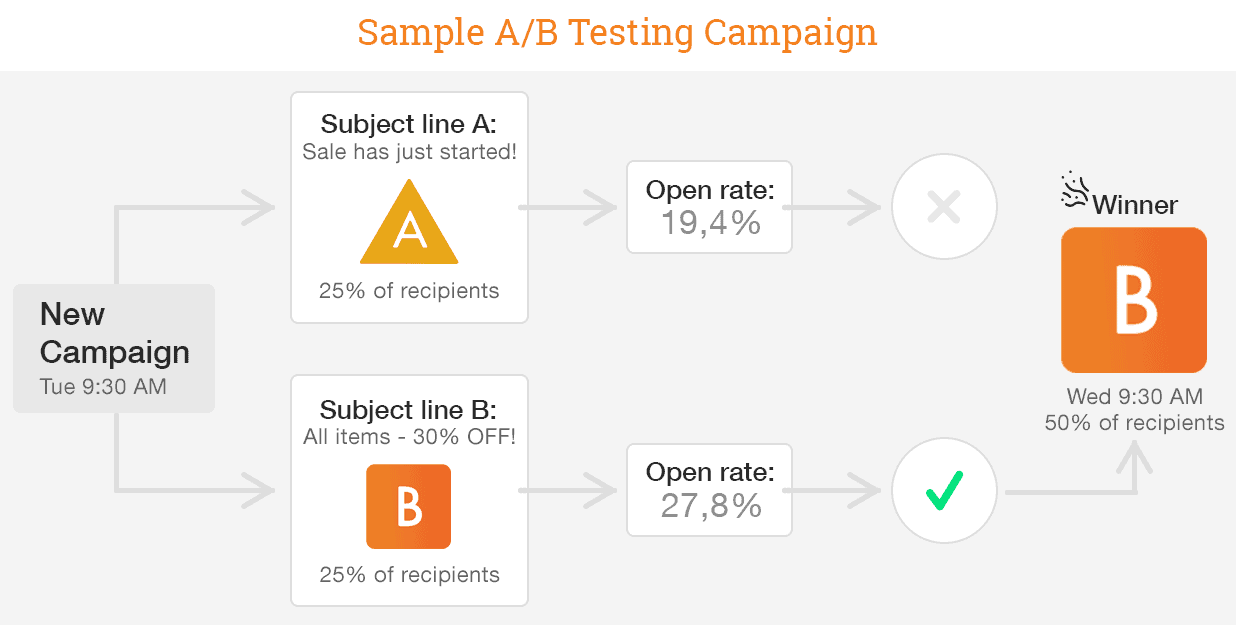
Imagine you have an email list of 10,000 people and you want to send an email out. You have 2 headlines and you are not sure which one would have a better open rate.
A common practice for email would be:
- Create 2 variations of headline: A & B
- Everything else remains the same
- Randomly choose 1000 people as an experiment testbed and split 50-50 (500 people each).
Conversion in this experiment: Open rate
Outcome: The winning headline will be sent to the remaining 9000 people
Email Click-Through-Rate (CTR)
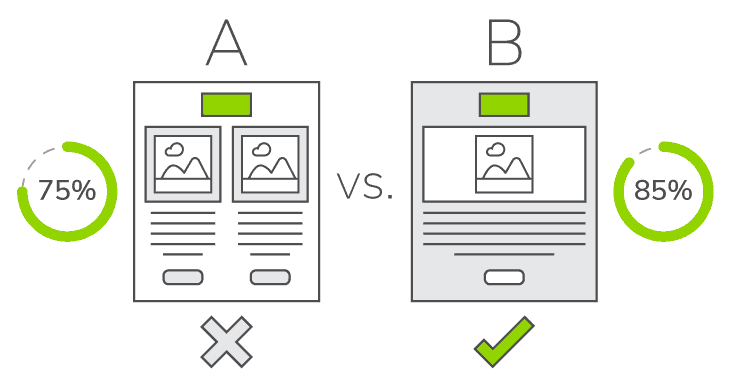
Imagine you have an email list of 10,000 people. You have 2 email copies and you are not sure which one would have a better CTR.
A common practice for email would be:
- Create 2 variations of content: A & B
- Everything else remains the same
- Randomly choose 1000 people as an experiment testbed and split 50-50 (500 people each).
Conversion in this experiment: Click-through-rate
Outcome: The winning content will be sent to the remaining 9000 people
Landing Page
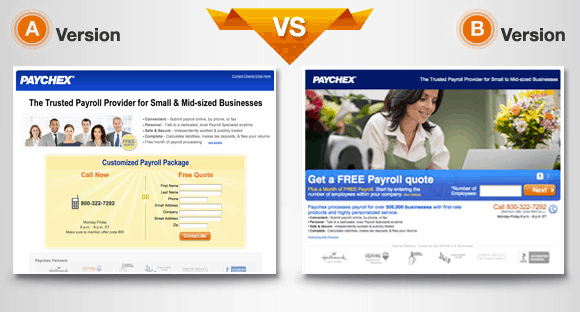
Similarly to the above examples, you can create multiple versions of the elements you want to test.
Common test:
- Headlines
- Pricing
- Visuals
- Testimonials
- Videos
- Form
Common conversion goals:
- Sign up rate
- Purchase
- Conversion rate
- Time spent on page
Outcome: The winning element will become a permanent fixture on the page
Facebook is similar as the above and it even has the A/B testing feature for you
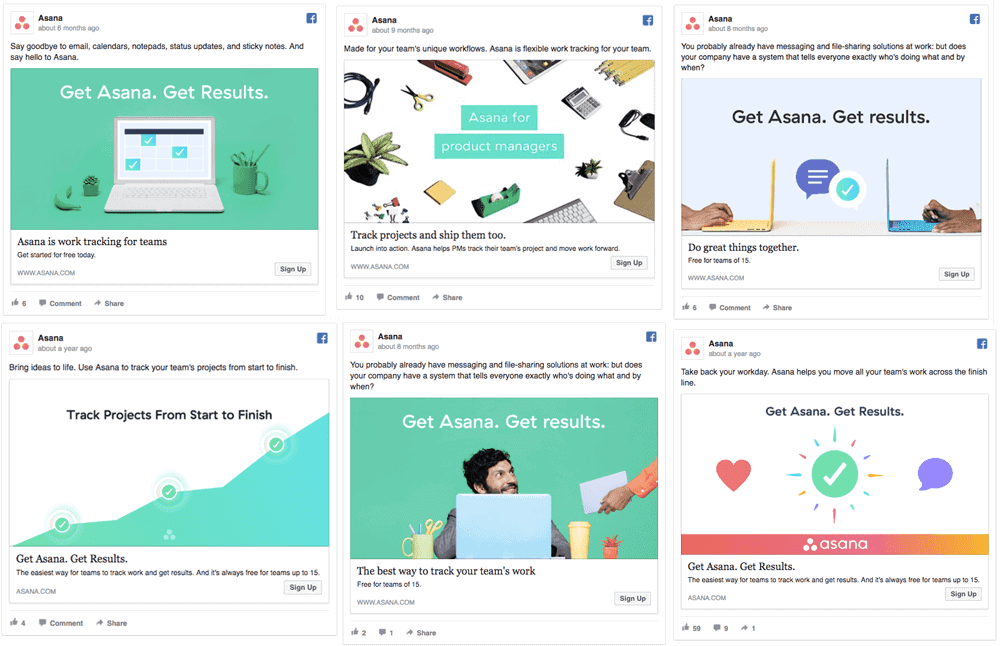
Common test:
- Audience
- Captions
- Headlines
- Placement
- Ad Creative
Common conversion goals:
- Click-through-rate to landing page
- Sign up rate
- Purchase conversion rate
A/B Testing Tools
Doing an A/B test requires you to have proper tools.
Email A/B testing tools
- Most email softwares have this tools in place
Advertising platforms A/B testing tools
- Most advertising platforms such as Facebook, Instagram, Google Ads enable you to do A/B testing or have functionalities similar to that
Landing Page A/B testing tools
- Optimizely: Paid
- Google Optimize: Free
- VWO: Paid
Type of test: A/B Testing, Multivariate testing, split testing
A/B Testing, Multivariate testing, split testing
There are several types of test which you may have heard of:
A/B testing
- Change 1 element while everything else remains constant
Multivariate testing
- Change more than 1 element and the program will do different combinations of the elements to achieve the best result
- Assuming you have 3 versions of headlines and 3 versions of visuals, there would be a 9 (3×3) combinations for testing
Split testing
- Test on 2 completely different variations all together
When not to do A/B Testing
While A/B testing is great fun, here are some things to look out for to ensure your marketing experiment is a success!
- Not enough traffic & conversions
If you do not have meaningful traffic, you would not be able to even run an experiment that would yield a conclusive result.
You would want your test to be statistically significant. Use this tool to check: https://neilpatel.com/ab-testing-calculator/
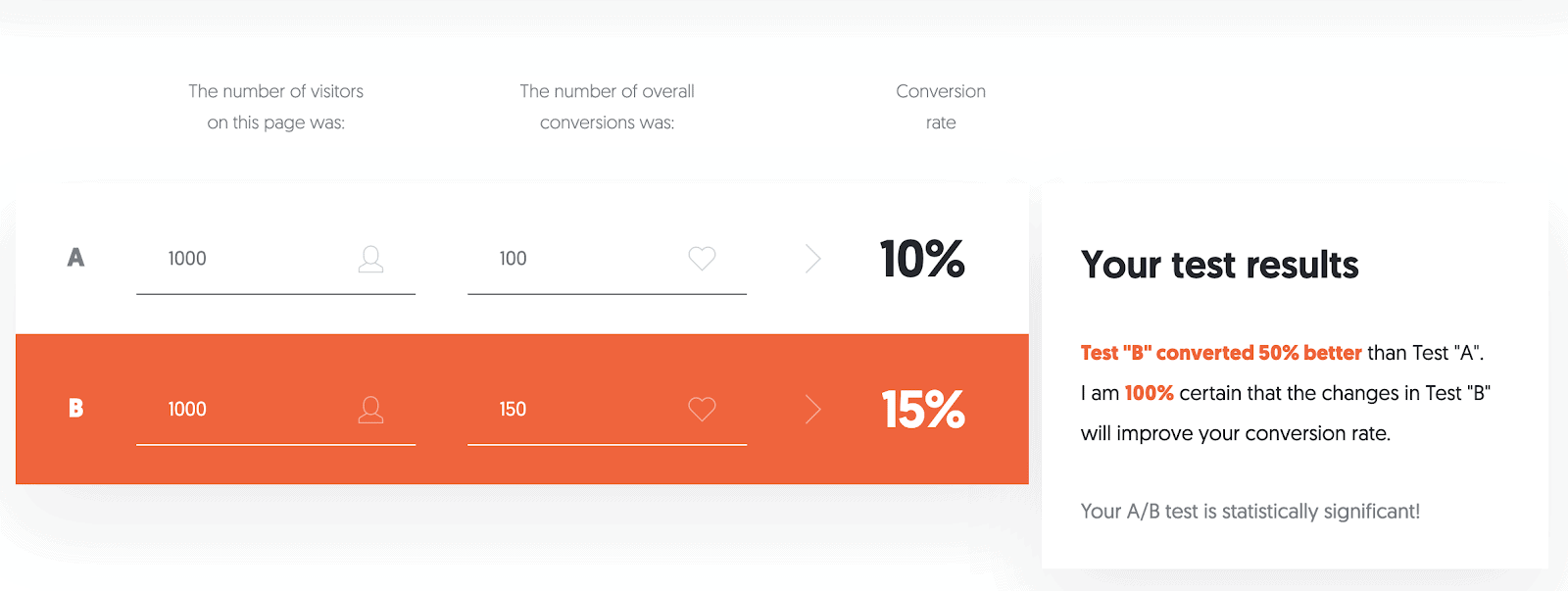
- No idea what you are testing for
If you don’t know what goals you are trying to achieve, it would be pointless to run an A/B test. You should also make sure the conversion goal makes sense.
For example: If you are testing email headlines, the goal should be open rate, not email click-through-rate.
- Not planning to take action
If you are not planning to take any action at all, save your time and do something else instead. π
A/B Testing Statistics & Data Science
Now that you know the concept behind A/B testing, it is time for the science behind it!
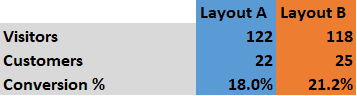
According to towardsdatascience.com,A/B Testing is similar to hypothesis testing.
Here are a few things to keep in mind:
- Forming a hypothesis
- Sample size and randomization strategy
- Method of measurement
Forming a hypothesisΒ
Example:
- Null hypothesis (H0):Β
- Nextacademy.com visitors that receive Landing Page B will not have higher lead conversion rates compares to visitors that receive Landing Page A
- Alternative hypothesis (H1):
- Nextacademy.com visitors that receive Landing Page B will have higher lead conversion rates compared to visitors that receive Landing Page A
Notice that it should follow this pattern:
- Population: visitors of NEXT Academy
- Intervention: new landing page B
- Comparison: landing page A
- Outcome: Lead conversion rate
- Time: Completion of a session on NEXT Academy’s website
Randomization strategy
There are several randomization and cluster strategies available, but in this case, many of the tools such as Google Optimize or Email softwares do a random sampling strategy.
For example:
Within the week, there are 10,000 visitors to your website and the software will randomly distribute the people.
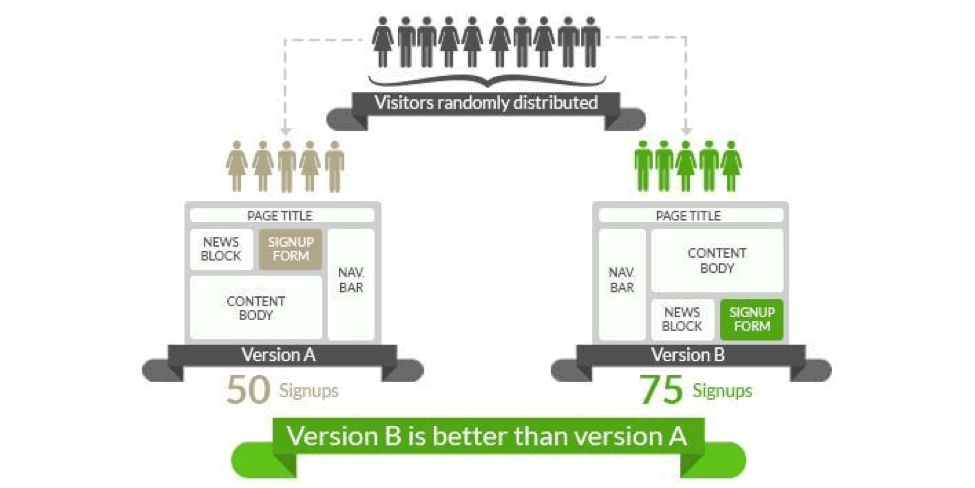
Why should we randomise?
- To distribute co-variates evenly – such as gender, location etc.
- To eliminate statistical bias – often we assume the sample is representative of our entire target population. If your sample is handpicked without much consideration, you would make a wrong conclusion to your entire target population, in this case, the visitors of NEXT Academy.
Sample Size
You can’t be taking a sample size of 10 people and assume it to be true for 10,000 people right?
You may have heard of p-value.
P-value means “In statistical hypothesis testing, the p-value or probability value is the probability of obtaining test results at least as extreme as the results actually observed during the test, assuming that the null hypothesis is correct.”
In other words, how statistically significant is the result. The more significant, the higher the probability that the result holds actionable recommendations.
Look at the 2 examples below. Both have the same conversion rates.
- The one with 2000 visitors has 99% certainty and it is statistically significant
- The one with 200 visitors only has 76% certainty and it is not statistically significant
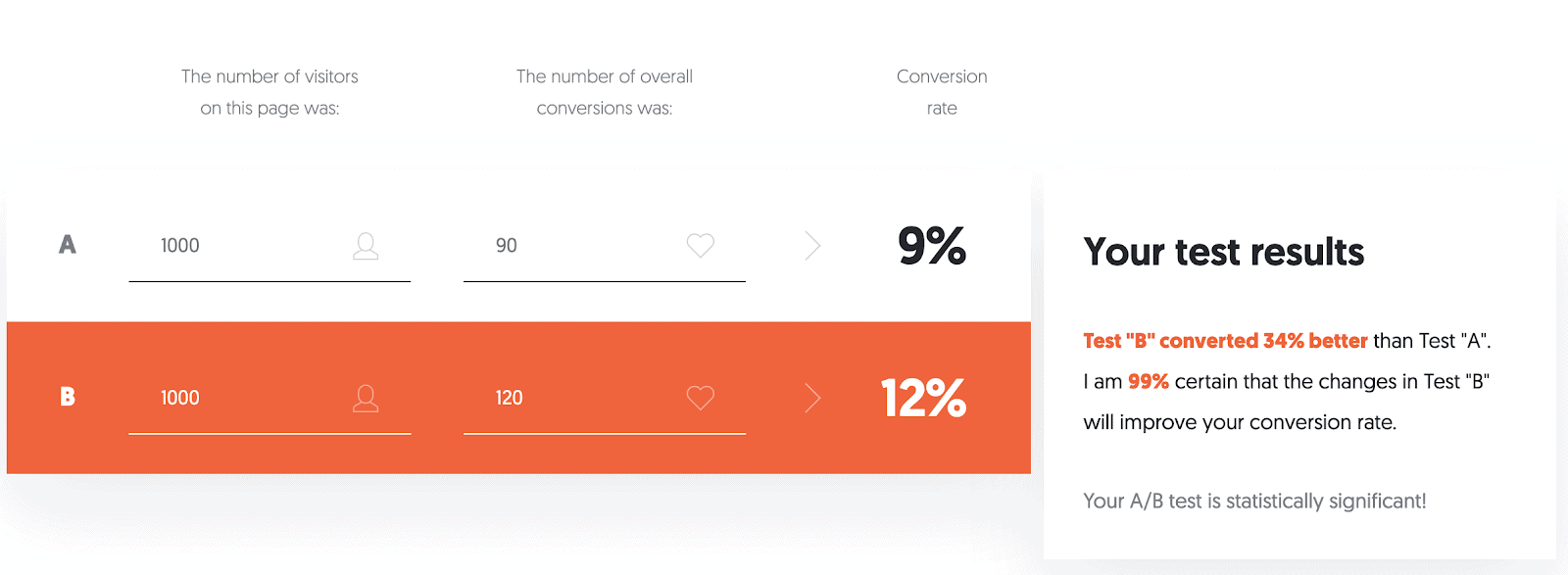
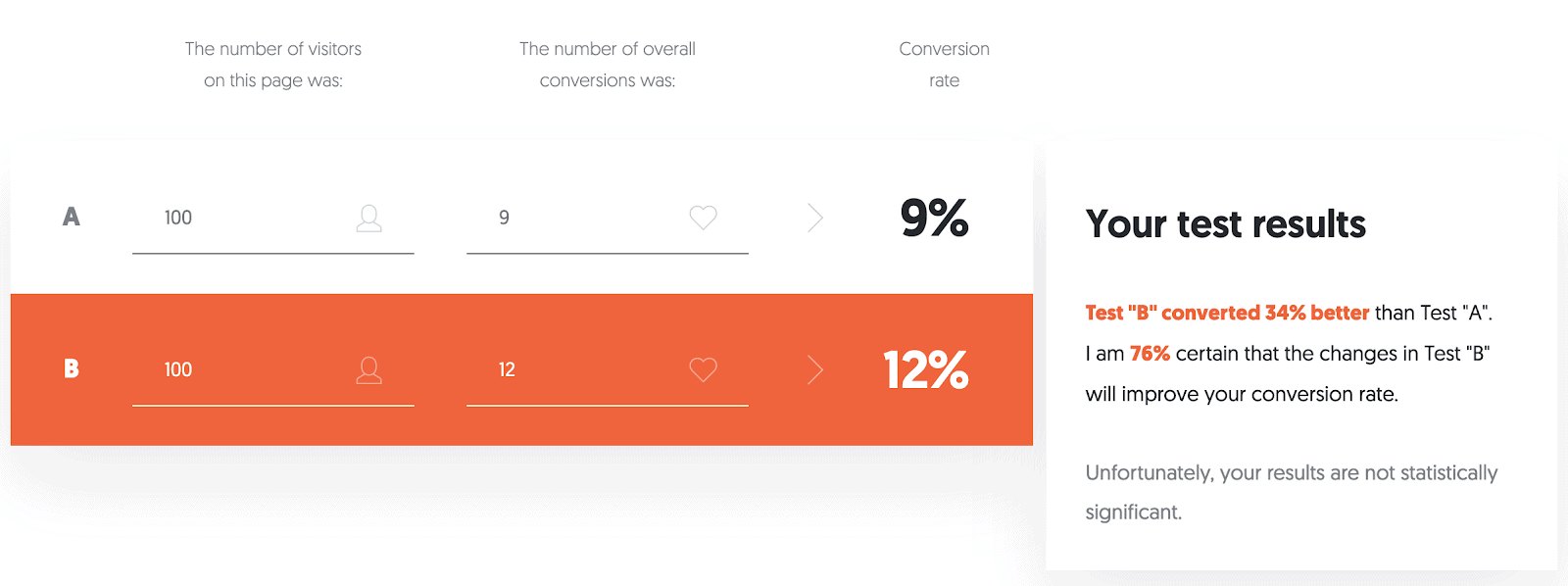
Often, you do not have control over the number of people coming into the website or what not.
How does softwares such as Facebook determine significance?
Tools such as Facebook Ads would have a learning period where they would want to obtain at least 50 conversions within 7-days to be able to safely conclude which ad is truly working before showcasing the result to you OR optimising the ads for you.
Measurement
Measure the wrong thing and your test would not make sense.
Let’s just say that my landing pages are poised to collect leads, but what happens if I ended up using “purchase” as my measurement for success?
Understanding the test design is important, here is an example:
- Visitors (same source, randomly distributed)
- β Landing Page A or Landing Page B
- β Lead (influenced by the Landing Page they landed on)
- β Salesperson would call
- β Purchase (influenced by Landing Page + Salesperson)
This would mean that by me using Purchase as a measurement of success would not be reflective on the test because there are added variables that have influenced the outcome.
In Conclusion
A/B testing is a powerful methodology and tools have made it simple for us to execute and take actionable moves for marketing purposes.
Nonetheless, understanding the statistics and data science behind A/B testing would open up ideas on how you can better test your market.
Just one thing though, these A/B testing activities require you to have good amount of traffic to make it work.
-
Josh Tenghttps://www.nextacademy.com/author/josh/
-
Josh Tenghttps://www.nextacademy.com/author/josh/
-
Josh Tenghttps://www.nextacademy.com/author/josh/
-
Josh Tenghttps://www.nextacademy.com/author/josh/